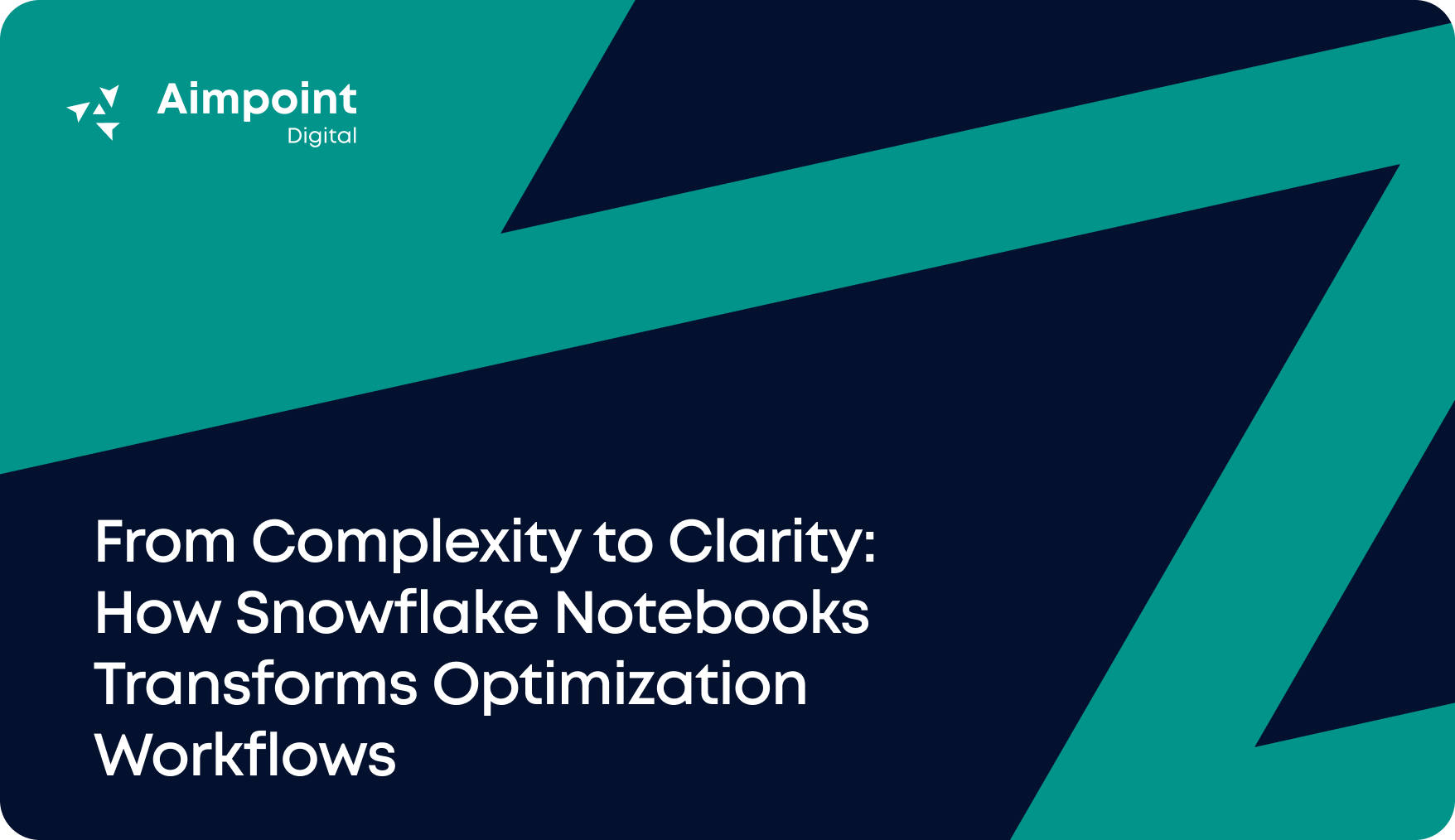
Optimization & Simulation
Data analytics is revolutionizing many industries, including manufacturing. Manufacturers have a tremendous opportunity to holistically analyze all data from the procurement, production, and distribution processes. While the amount and variety of data pose significant challenges, the potential gains for manufacturers in boosting overall productivity and profitability can be massive. All manufacturers should prioritize integrating modern analytics infrastructure that can distill complex data into actionable insights in real-time. Those that can effectively integrate manufacturing analytics into their organization will generate significant competitive advantages, while those that delay adoption run the risk of falling behind.
Manufacturing analytics is a broad term that encompasses various methods capable of transforming data into insights that then can drive desirable business outcomes.
The key elements common to these methods are:
Below, we highlight seven ways in which data analytics is transforming the manufacturing industry.
Discrete event simulation (DES) is a powerful tool for representing the increasingly complex modern manufacturing processes. DES models characterize processes as sequences of events occurring at discrete time points. Essentially, this recreates a factory in the virtual world. Discrete event simulations are versatile with various applications such as scheduling, capacity planning, resource allocation, bottleneck identification, and scenario analysis.
As an example, our team at Aimpoint Digital harnessed the power of discrete event simulations to accurately characterize uncertainty in the batch processing times of a major North American steel manufacturer. With a handle on this uncertainty, our team generated optimal production schedules for projected improvements in productivity of up to 25%.
Product quality analytics is the targeted application of data analytics techniques to improve the attributes of a product. Supplying high-quality products and services is the pillar on which the long-term success of any business is based. Quality checks can be sped up with product quality analytics, and potential downstream quality issues can be identified to warn operators in advance. In addition, analytics around quality can aid operators in suggesting actions for troubleshooting and resolving issues.
For example, our project team holistically analyzed 612 unique production parameters across 12 machines at a plastics manufacturer. We developed a solution for our client using causal inference techniques by identifying the parameters’ impact on product quality. As a result, our client experienced a 22% reduction in scrapped parts, and consequently, a 15% increase in throughput.
Productivity analytics refers to the use of data analytics to ensure that a manufacturing facility runs as smoothly as possible. This includes avoiding factors such as unplanned machine downtime (when machines are idle) that significantly impact the profitability of a manufacturing enterprise. Other detrimental factors include inefficient use of machines or labor due to poor resource allocation. By employing analytics tools such as inventory and demand forecasting, simulation, and optimization, industries can run their facilities at maximum capacity.
For example, our team of optimization experts identified opportunities for boosting overall equipment effectiveness (OEE) for a chemical industry client. The client was facing significant changeover and setup times leading to reduced OEE. By integrating operational considerations in the production planning process, our solution enabled our client to meet all orders while minimizing changeover and setup times, leading to a massive jump in OEE from 63% to 89%.
Manufacturing throughput quantifies the amount of intermediate and/or finished goods a facility can produce in a fixed amount of time. A facility running smoothly with minimal or no unplanned downtimes could still be operating inefficiently. With increased data, manufacturers can now isolate inefficiencies and bottlenecks that are limiting throughput. Data analytics can help identify appropriate tweaks in production parameters to optimize operations. This has the added benefit of net output increasing with existing infrastructure without needing expensive capital investments. As an example, by systematically implementing throughput optimization, General Motors was able to save a whopping 2.1 billion dollars.
Manufacturing yield is the fraction of inputs into a process that is successfully transformed into high-quality finished goods. Variations in raw materials can lead to variability in production output. Certain industries such as chemicals, mining, or pharmaceuticals are especially susceptible to fluctuations in manufacturing yield. While hundreds of production parameters are available in the modern manufacturing plant, how to adjust them to maximize product yield is not always clear.
At Aimpoint Digital, we have developed an Explainable Manufacturing (EM) platform to quantify how each production parameter impacts manufacturing yield. The EM platform relies on causal inference techniques to suggest the productions variables thought to be most impactful. The tool can analyze all data related to input material across facility machines and tell operators how changing each of those input variables will affect the machine throughput.
A production schedule defines the sequence of processes to execute on a material and equipment level to produce finished goods.
Operators often use rule-based heuristics or spreadsheet-based methods for their simplicity, but they can be suboptimal. On the other hand, mathematical optimization-based methods can guarantee optimal schedules. However, they may take longer computational times for convergence, making it challenging to implement them in real-time. Data analytics can bridge the gap in developing production schedules. By supplementing operators’ domain knowledge with data analytics, production schedules generally outperform rule-based heuristics. In addition, these techniques are efficient and generate solutions fast, making near real-time implementation even easier. For example, Dow Chemical Company is exploring the use of deep reinforcement learning for scheduling chemical plant operations.
Predictive maintenance techniques allow operations to go beyond static maintenance schedules by incorporating data to gauge machine health and predict when a machine may need upkeep.
Machine and equipment failure have high financial and business costs. Many industries employ periodic maintenance schedules, but these are static. They do not factor variances in machine health deterioration. For example, some machines may need more frequent maintenance, while other machines could faithfully function with delayed maintenance schedules. Machine sensor data combined with statistical and artificial intelligence methods can predict the probability of a machine breaking down. Using methods like time-to-failure analysis can help schedule machine maintenance dynamically. This will prevent failures, minimize interruptions, and maximize plant productivity.
At Aimpoint Digital, our mathematics experts continue to develop fundamental theories and solution algorithms for this area, including survival analysis.
Aimpoint Digital is committed to helping all industries, big and small, unlock the potential of data analytics to transform their manufacturing processes. In addition to knowledge of data science methodology, our team has fundamental knowledge and experience with a wide variety of engineering fields, spanning industrial, mechanical, chemical, nuclear, and biomedical. We bring a unique combination of manufacturing domain expertise and data and analytics skills to address challenges facing manufacturers.
Contact us to learn how to exploit data to improve your manufacturing processes.
Whether you need advanced AI solutions, strategic data expertise, or tailored insights, our team is here to help.