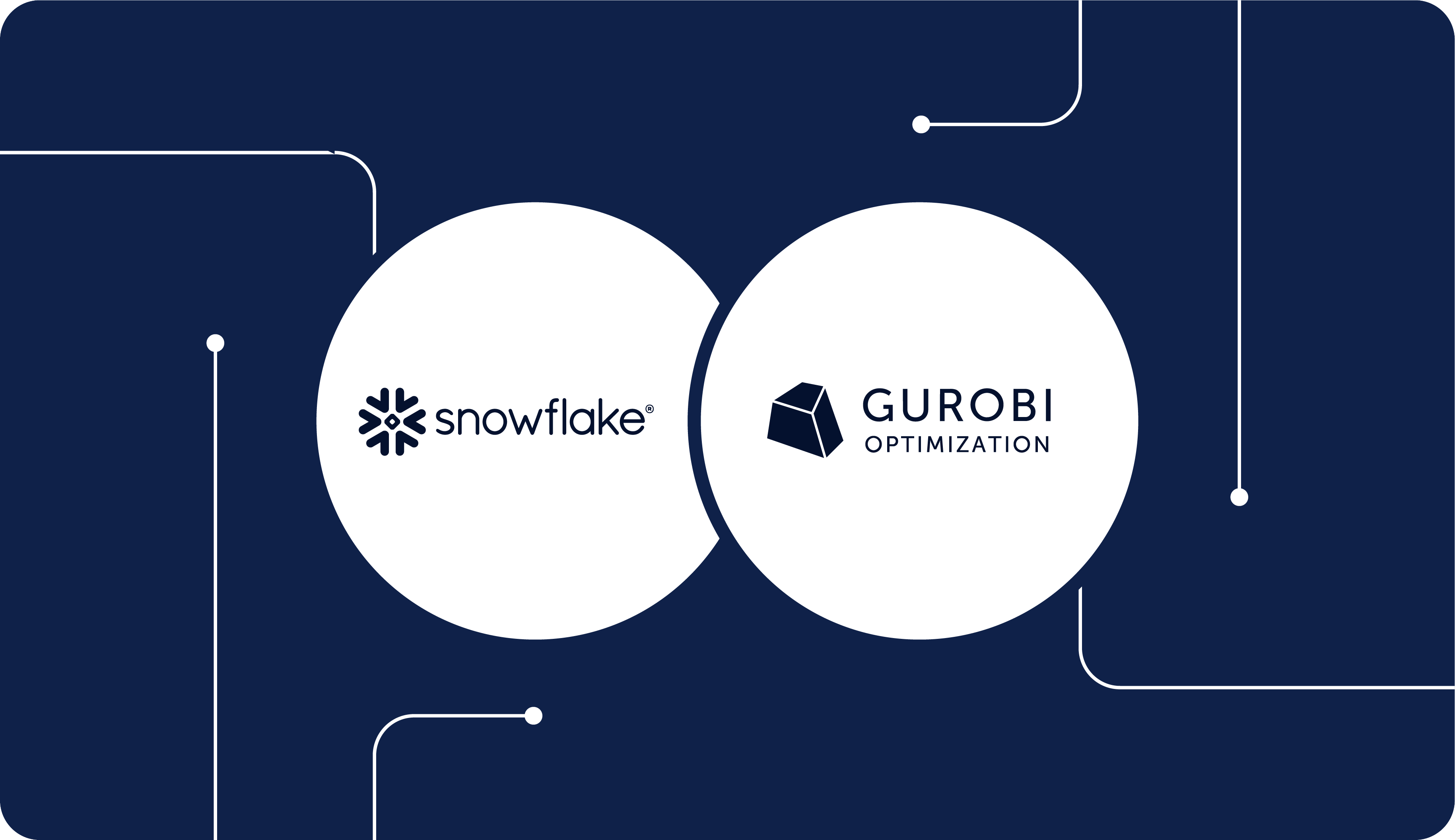
Optimization & Simulation
Product quality analytics are of vital importance to all manufacturing industries. The ease and availability of inexpensive sensors and data storage have led to an explosion in data availability. The continued emergence of new technologies further enhances connectivity and the speed at which data becomes available. These advancements provide unique opportunities to apply product quality analytics to maximize throughput.
In manufacturing, inferior quality products run the risk of reducing customer satisfaction and lost sales. Their consequences can sometimes be catastrophic. Suboptimal production processes lead to productivity losses. Products that do not meet quality standards must be discarded, but that is not all that is lost. The raw materials, machine time, and labor time that produce these substandard products are also often wasted. Thus, the long-term success of any company relies on the high quality of the goods and services it provides.
The collection of larger quantities and new types of production data at higher resolutions, combined with modern machine learning techniques, provide opportunities to improve production further. Product quality analytics helps boost production in the following ways:
Product quality analytics analyzes production data and provides predictions and recommendations in real-time. This significantly expands the value of production quality analytics in maximizing throughput over traditional methods that rely on the analysis of historical data.
A plastics industry client was tracking hundreds of features across multiple machines, each of which operated slightly differently. The client wanted to improve product quality by understanding how to change each of these features.
The project team at Aimpoint Digital applied causal inference techniques to provide both high-level and detailed insights into the drivers affecting product quality. Each of the 51 individual manufacturing machines has 12 available parameters or 612 settings in total. The constructed solution combines machine learning with causal inference and automation to simultaneously fine-tune these settings to ensure high-quality production. By leveraging dynamic nonlinear modeling techniques, our team determines the precise settings of these machine parameters across the entire facility, optimizing product quality.
Operators can now understand which machine features they needed to adjust to maximize quality, achieving a 22% reduction in scrapped or wasted parts. As a by-product, the reduction in scrapped parts and increased production has also improved throughput by 15%.
Increased data and advanced analytics have massive potential to improve product quality and production in the manufacturing sector. We can ensure the long-term benefits of advanced analytics for quality management and throughput optimization by ensuring that we design solutions with ease of deployment and maintainability in mind.
Contact us to learn how to exploit data to improve product quality.
Whether you need advanced AI solutions, strategic data expertise, or tailored insights, our team is here to help.