What are Analytics Engineers, and why does your organization need them? What differentiates them from the Data Engineers or Data Analysts you may already have on your data team? What pain points can they help alleviate for your business?
Businesses now have access to more data than ever, and decision-makers are demanding more from it. Successful organizations set themselves apart from competitors by fostering a data-driven culture and rapidly turning data into trustworthy, actionable insights. However, these efforts are often hampered by the incompatibility of various systems, lack of trust in data assets, and inefficiency bottlenecks. Business users frequently resort to being reactive rather than proactive - duplicating each other’s efforts and taking shortcuts that lead to future technical debt.
With the development of tools like dbt, Snowflake, and Databricks, which scale cost-effectively, modern data teams can help organizations avoid these pitfalls and get the most out of their data. Analytics Engineers are positioned between Data Engineers and Data Analysts and are focused on modeling and providing clean, trustworthy, reusable datasets to analysts and business users.
Imagine the modern data team as the staff running a large physical warehouse store like Costco. In this analogy, we can think of the Data Engineers as those responsible for operating and managing the trucks that deliver goods to the warehouse. The Data Analysts are like the front-facing staff, interfacing with customers to understand their needs and retrieving those items from the warehouse. The Analytics Engineers are like the warehouse floor managers who track inventory and decide how to organize the goods on the shelves to enhance retrieval efficiency and ease of access.
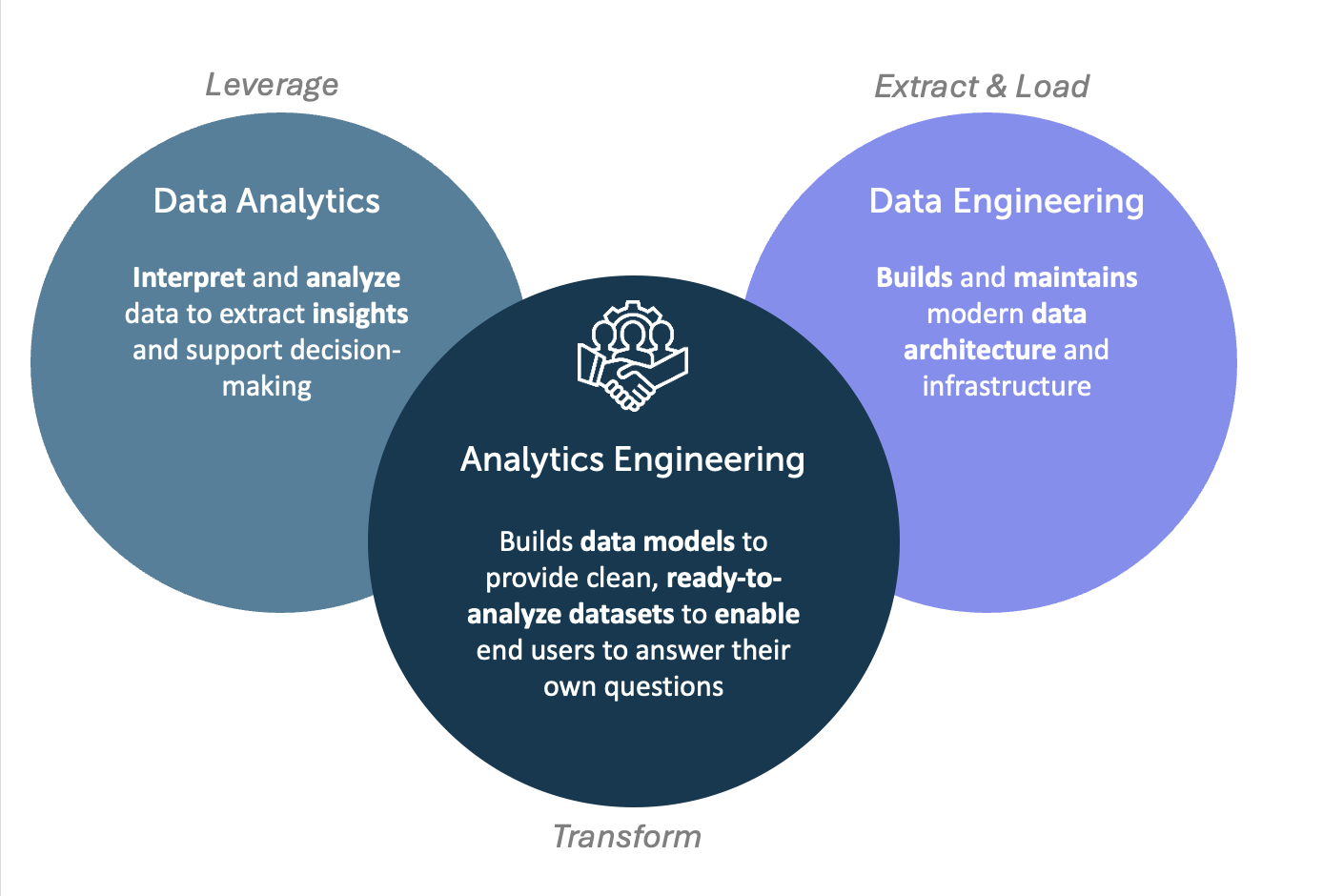
A great modern data team makes use of Data Engineers, Analytics Engineers, and Data Analysts – allowing each of them to do what they’re best at and taking full advantage of their unique skill sets.
Here are our six reasons why every organization needs Analytics Engineering:
- Boosts Operational Efficiency
- Sets the Foundation for a Data-Driven Culture
- Accelerates Innovation
- Clarifies Data Ownership and Accountability
- Enables Scalable Data Ecosystems
- Adapts to Modern Cloud Data Warehouses
Let’s dig into each of these in more detail below.
Boosts Operational Efficiency
As organizations of all sizes come to understand and rely on data to make business decisions, it becomes increasingly important that the bridge between business expertise and data expertise is well-defined. In practice, this bridge is often non-existent or, at best, delegated haphazardly to whoever on the existing data team happens to have free time. Let’s review a practical example to illustrate why this is inefficient and how Analytics Engineering solves each issue.
Lindsay, the CEO of a leading media and entertainment company, has a simple question she wants answered:
What’s the average difference in revenue between an event that occurs on a US holiday and an event that doesn’t?
To answer this question, Lindsay asks the Director of Data, Warren, to get back to her within a week. Warren then delegates this task to Amy, a Data Analyst who just finished her previous project and is looking for her next one. Over the next week, Amy runs into the following problems:
- Definitions: What did Warren mean by revenue? Does he mean net revenue or gross revenue? Is that before or after taxes? What about coupons? And what about holidays? Does Halloween count as a holiday? What about ethnic or religious holidays?
- Communication: Amy is waiting to hear back from Warren about these questions, who himself is waiting to hear back from Lindsay. Amy is also waiting to hear back from the data engineering team, who pointed Amy to where they think the relevant data is stored in the data warehouse.
- Role creep: As a Data Analyst, Amy has never had to navigate through a data warehouse. Typically, the data engineering team provides a curated dataset ready for analysis.
A lack of definitions, unclear communication pathways, and role creep are issues that lead to inconsistent and incorrect answers, untimely delivery, a lack of trust, and employees operating outside of their area of expertise.
How does Analytics Engineering address these problems and ultimately increase business efficiency?
- Definitions: The Analytics Engineering team already has a single source of truth for Amy to use. With tool features like dbt’s semantic layer acting as a company-wide data dictionary, there is no need for Amy to go on a data treasure hunt or to produce her own local definitions like revenue and holiday.
- Communication: As Director of Data, Warren knows to consult the Analytics Engineering team to advise whether the data to answer Lindsay’s question is already available. Like a librarian who knows which books are available and where they are located, the Analytics Engineering team is responsible for organizing and documenting assets in the data warehouse for analysts to use.
- Role Creep: Analytics Engineers are hired to bridge the gap between Data Engineers and Data Analysts. Analytics Engineers enable Data Engineers to focus on data architecture, data ingestion, and performance optimization and allow Data Analysts to focus on extracting insights and building reports.
With Analytics Engineering, ambiguous requests are quickly transformed into action, saving time for executives and the data team.

Sets the Foundation for a Data-Driven Culture
When executives want to make informed decisions to drive their business to new heights, how do they do that? How does an organization value data and engrain in its employees a data-driven culture where strategic decisions make sense to a senior-level executive and a newly hired Data Analyst? Lastly, how does Analytics Engineering contribute to such a culture?
At the root of a data-driven culture is trust. With trust in the numbers and trust in the process that led to those numbers, an organization is set up to make data-driven decisions confidently. Without this trust, confidence in the data team and their outputs erodes, and organizations quickly fall back into the old days of operating on instinct.
Let’s look at how Analytics Engineers build trust through documentation and data quality.
To an organization’s peril, data documentation is avoided. It’s thought of as boring and tedious. Besides, who actually reads the documentation?
Analytics Engineering takes the documentation and evangelization of data assets as a primary focus. An organized data warehouse or Lakehouse makes data more available, enables self-service, and reduces the need for meetings between the business and the data team. Fortunately, Analytics Engineers can leverage tool features like documentation generation and graphic data lineage DAGs (directed acyclic graphs) from dbt, or data lineage with Unity Catalog from Databricks - enabling anyone to see which data sources contributed to each calculation or table, see figures below.


With this level of transparency, it’s much easier for the end users of data to make sure that the numbers they are seeing are calculated correctly. Additionally, with the “data recipe” available for all to see, any mistakes or missing context are easily found and remedied.
Businesses have rules, and while they vary in how they appear in the data, one common, illustrative example is the notion of an ID column. Any ID column should only map to a single product, user, transaction, etc. If this rule is broken, downstream calculations and reporting metrics break. Without accurate data, trust erodes, and the business loses out on making accurate decisions. Modern data tools like Databricks and dbt allow Analytics Engineers to set constraints on these ID columns to ensure that an ID column only ever maps to a single product, user, or transaction, providing peace of mind that business rules are enforced down to the appropriate level.
Accelerates Innovation
One type of question that plagues traditional data teams is the inability to answer a key question quickly: Can we run an analysis request or build a new data product with existing data assets, or do we need to ingest new data or build a net new pipeline?
As custodians of curated data, Analytics Engineers can answer these art of the possible type questions. Returning to our example in the “Boosts Operational Efficiency” section, Amy did not know whether a definition for holidays existed. The Analytics Engineering team would direct Amy to the holidays table, generally providing direction to analysts. Furthermore, if a calculation needs tweaking or a new pipeline needs building, the Analytics Engineering team ensures a quick and reliable turnaround anchored by a key asset: the data model.
With an organized and documented data model (which Analytics Engineers specialize in producing - you can read more about Aimpoint Digital’s guide to effective Data Modeling here), Data Analysts can more easily and quickly produce essential business insights. In short, a data model acts as a kind of map for data analysts to use to understand where the data lives and how data assets are related to each other. With a data model, Analytics Engineers reduce the time analysts spend locating and building a curated dataset, allowing them to focus on high-value activities like identifying trends, discovering new opportunities and making data-driven decisions.
As businesses evolve, updates to their data definitions and pipelines are inevitable: new products are added, novel categories are formulated, and state-of-the-art data products are required to maintain a competitive advantage.
Previously, without a centralized transformation layer, each report needed to be individually updated, which was a painstaking, error-prone, and lengthy process. Now, with a centralized data model, only one place needs updating. Adhering to continuous integration and continuous delivery (CI/CD), Analytics Engineers ensure that changes to the data model are quick and painless as needs arise. This fast and reliable development cycle encourages new ideas from the business, accelerating innovation.
Clarifies Data Ownership and Accountability
Inevitably, things break, and there is no difference when it comes to reports and data products. One point that separates great teams from good ones is that great teams clearly delineate responsibility. If the data ingestion breaks, the Data Engineer troubleshoots it. If the report breaks, the Data Analyst comes to the rescue.
What happens if one of the steps between ingestion and report within the transformation layer breaks? From Aimpoint Digital’s perspective, the Analytics Engineering team is accountable for the transformation layer. Adding this extra layer of ownership solidifies responsibility across the data team.
Modern tools like dbt aid in the automatic creation of DAGs (directed acyclic graphs). Pictured below, a DAG shows the flow of data from source to report, making it easy to know where to look when data assets break and who to contact for support.
DAGs also help the business understand which downstream processes are affected if a source table is updated or removed. Analytics Engineers will be the masters of modern data ETL tools like dbt, Databricks’ Delta Live Tables feature, and Sigma Computing’s Dataset and Data Models features, and having one on your team means you’ll be able to leverage the full range of capabilities of these tools.

In addition to owning the transformation layer (i.e. data model), the Analytics Engineer is responsible for evangelizing the data model so that downstream users like analysts and data scientists are aware of the available data and how it’s organized. Hosting demos ensures that consumers of the data model know where to look for relevant data, how to use it, and its limitations. Educating all downstream users on the transformation/data model layer serves another purpose: enabling scalable data ecosystems. Let’s take a closer look.
Enables Scalable Data Ecosystems
Within their data systems, organizations struggle with internal consistency. If Bill’s report claims $83,000 in sales, but Angela’s says $70,000, who’s right? How can leaders confidently make decisions if they do not know which data are correct? Moreover, Bill and Angela’s incongruent sales totals point to another issue: the duplication of effort.
Bill and Angela worked independently to calculate this sales number. Otherwise, their numbers would match. If only there were a way to guarantee consistent results and to reduce redundant efforts throughout the organization.
A core value proposition of analytics engineering is to centralize the transformation layer out of existing BI platforms, thereby reducing data siloes, creating a single source of truth, and keeping data assets organized and up to date. As proprietors of the transformation layer, Data Analysts, Data Scientists, and business users have a dedicated resource when a number looks off, or when updates need to be made to existing business logic. Analytics engineering helps organizations scale their data ecosystems by reducing the proliferation of ad-hoc, untested pipelines and queries.
In addition to internal consistency, analytics engineering provides a structure for novel business questions to pass through. Suppose a new business request or question is similar to one already being asked and answered. In that case, the analytics engineering department, with centralized knowledge of all existing pipelines, is positioned to quickly decide if a net new pipeline or query is necessary or whether a slight adjustment to an existing asset is all that’s needed. This reduces the turnaround and unnecessary effort for the data team.
Finally, data ecosystems stumble when, inevitably, data professionals move on from an organization. The extent to which they stumble, however, is largely a matter of how much tribal knowledge that individual kept to themselves. With analytics engineering’s focus on documentation and evangelization, more of the data team understands the existing inner workings of data models and pipelines. This AE-driven data outreach, through documentation and demos, hedges against those single-point-of-failure individuals.
Adapts to Modern Cloud Data Warehouses
With companies adopting modern cloud data solutions like Snowflake and Databricks, increasing amounts of data are becoming available to the business. If left unmanaged, this data warehouse, or “Lakehouse” for those keeping up with the latest slang, can quickly become a data swamp with too much data, too little documentation, and too little value to the business. The cost of maintaining a data swamp is two-fold: one, duplicate data and redundant pipelines incur higher storage and compute costs, and two, data users spend more time navigating the warehouse for relevant and reliable data. Analytics Engineers take on the responsibility of organizing the data warehouse, optimizing pipelines, reducing tech debt, and building only what needs to be built.
In addition to data warehouses containing more data than ever before, data warehouses are also evolving into platforms that Data Engineers, Data Analysts, Machine Learning Engineers, and Data Scientists use to accomplish their day-to-day responsibilities. As data volumes and the number of folks who use these platforms grow, the importance of keeping the warehouse organized for each of these roles emerges as a new priority.
Despite its title, “analytics engineering” is more than engineering for analysts. Machine Learning Engineers and Data Scientists also consume data in the warehouse. Remember, with platforms like Databricks offering a one-stop-shop for all things AI and BI, these formerly disparate teams have a better opportunity and more integrated platform in which to collaborate. This means that Analytics Engineers can set up a set of data models for the Data Analysts and Data Scientists. These models will vary in complexity, rawness, and level of aggregation, but all will come with the advantages that analytics engineering promises: documentation, reliability, and accountability.
Conclusion
As the volume and complexity of data available to organizations grows, Analytics Engineers will become increasingly valuable assets to modern data teams. It’s more important than ever for businesses to access reliable and robust datasets, data pipelines, and data models to harness their data’s full potential and derive actionable insights from them.
Analytics Engineers are ideally positioned to help your business make these data-driven decisions more quickly and confidently while helping avoid operational bottlenecks and inefficiencies. Simply put, adding an Analytics Engineer to your team will increase the effectiveness of your data team’s operations and help lay the groundwork for a data-driven culture that supports strategic growth and innovation.
At Aimpoint Digital, our team partners with organizations of all sizes to enable self-service analytics or to crack that particularly difficult use case. If you would like to learn more about our services and offerings or would like to share any feedback with us, please do not hesitate to contact our growing team of experts.